Prof. Aleksandra Kawala-Sterniuk from the BANANA team, Polish partner, visited on 14-16.06.2023 Torun.
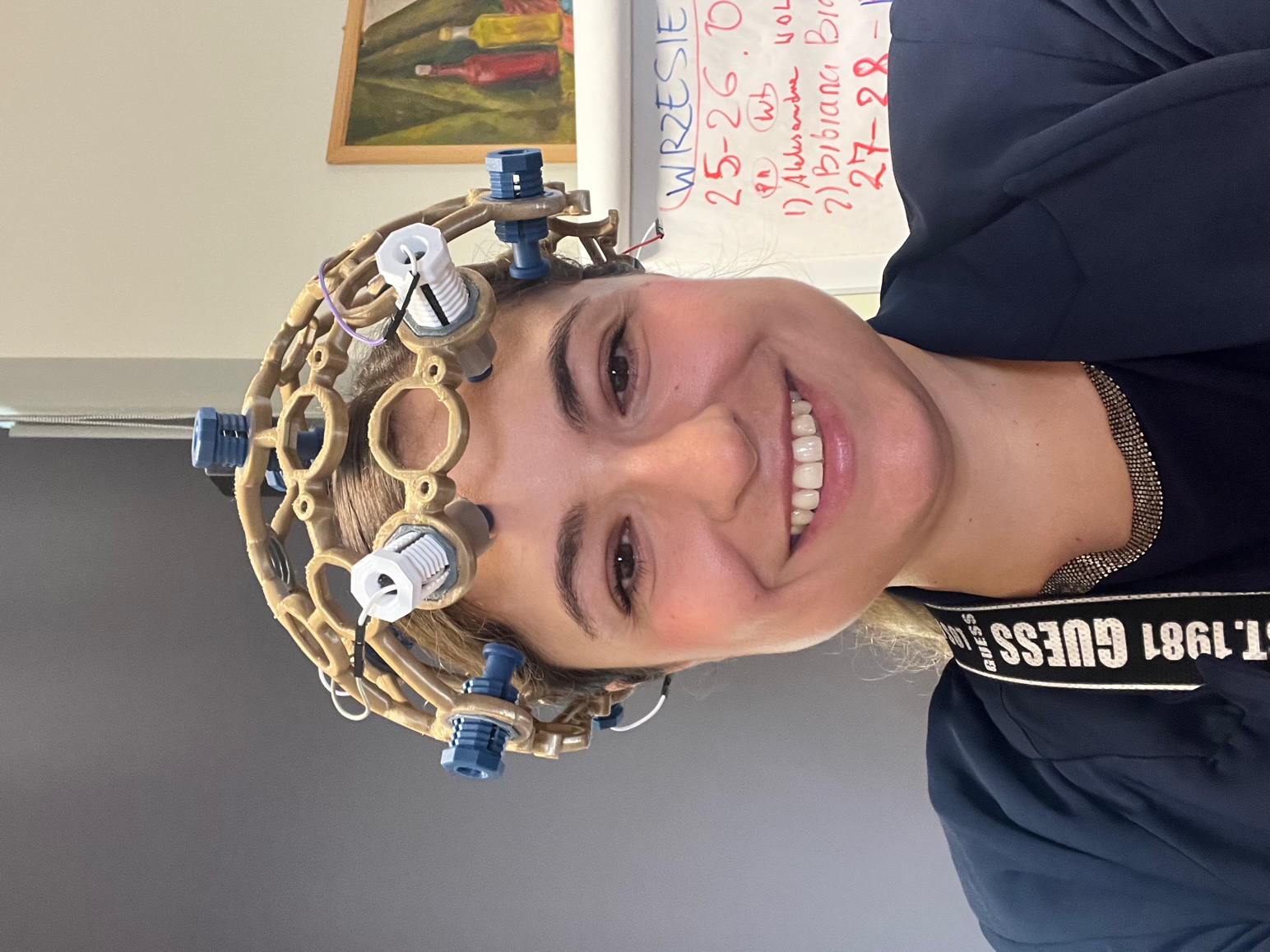
She met Polish-PI and her team from BITSCOPE (Brain Integrated Tagging for Socially Curated Online Personalised Experiences) – prof. Veslava Osinska from the Nicolaus Copernicus University in Toruń (Poland).
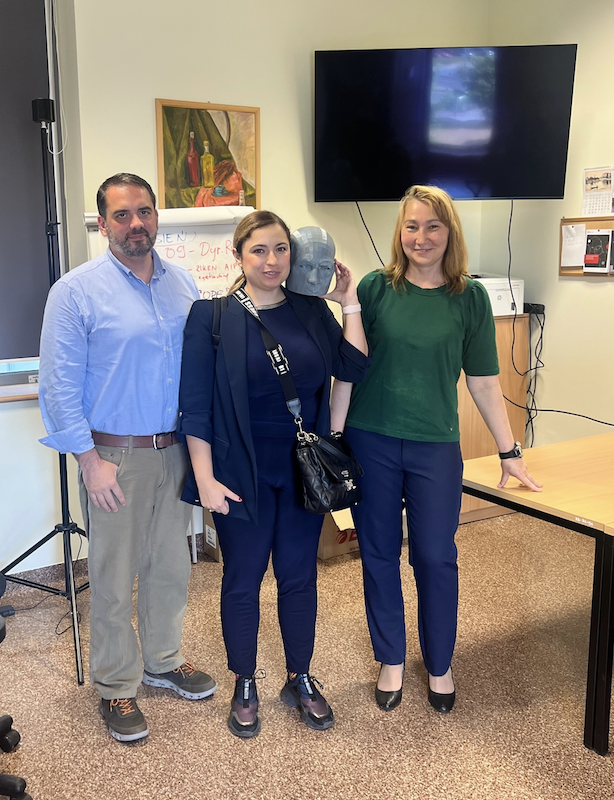
BITSCOPE introduces a vision for enhancing social relationships through brain-computer interfaces (BCIs) in virtual environments. Instead of relying on explicit feedback like “likes,” our improved BCI technology captures attention, memorability, and curiosity by passively collecting neural data signatures. This data, refined through machine learning, can be used by recommender systems to create better online experiences. Our work focuses on developing a passive hybrid BCI (phBCI) that combines electroencephalography, eye tracking, galvanic skin response, heart rate, and movement to estimate the user’s mental state without interrupting their immersion. This approach improves signal quality, denoising capabilities, and adaptability to home environments. We leverage deep learning, geometrical approaches, and large datasets to address user state classification, including attention, curiosity, and memorability. These advancements are achieved through co-designed user-centered experiments.
BITSCOPE partners:
- Dublin City University – Ireland (Coordinator)
- Universitat Politècnica de València – Spain
- Centre de Recherche Inria Bordeaux – Sud-Ouest – France
- Nicolaus Copernicus University – Poland
She and Dariusz Mikolajewski (Co-PI, OUT) also had a pleasure to meet prof. Dean J. Krusienski from VCU, leader of the ASPEN Lab.
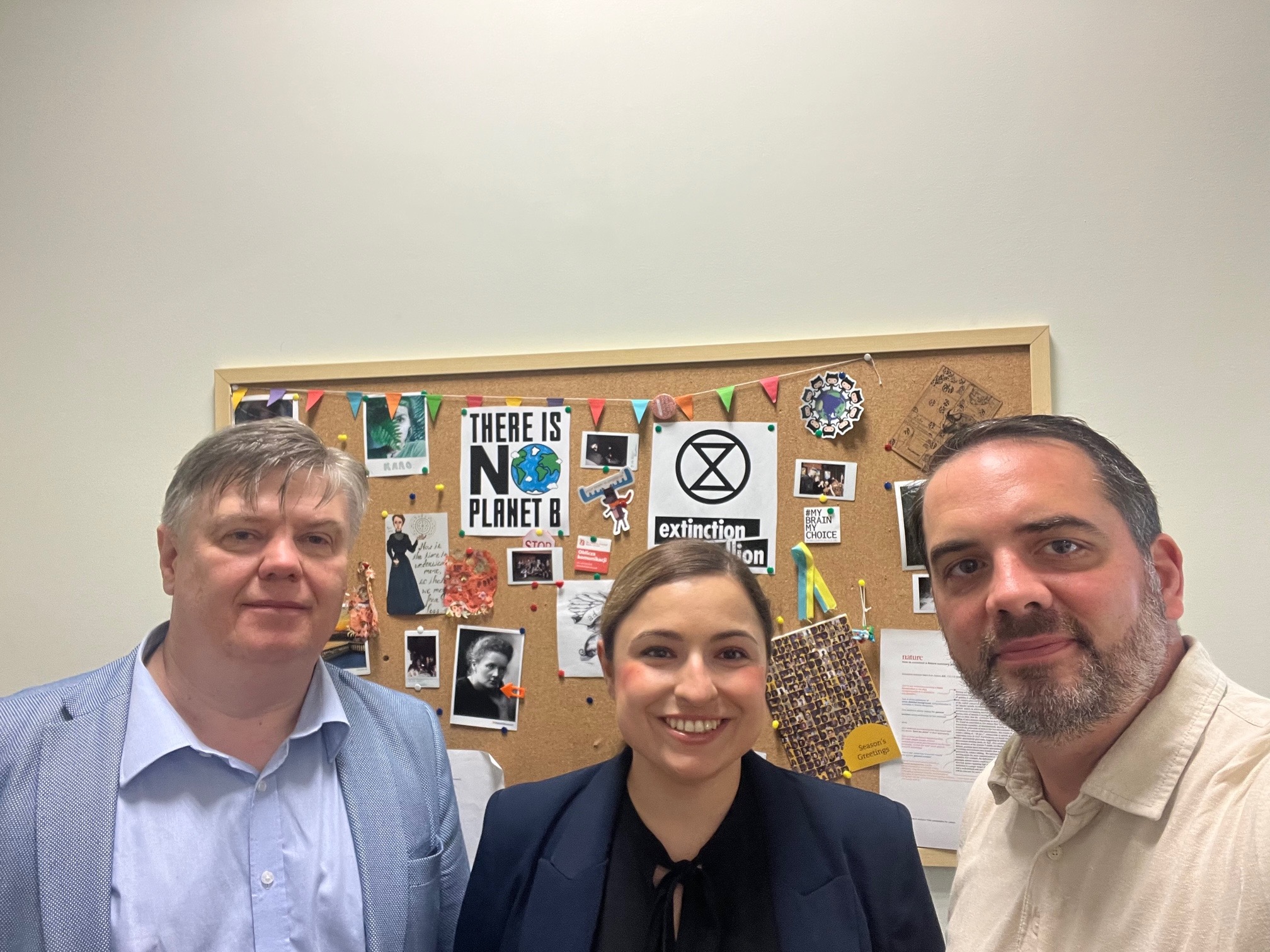
Dean J. Krusienski, a Senior Member of IEEE, obtained his B.S., M.S., and Ph.D. degrees in electrical engineering from The Pennsylvania State University, University Park, PA, USA. He conducted his Postdoctoral Research at the Brain-Computer Interface Laboratory, Wadsworth Center of the New York State Department of Health. Currently, he holds the position of Professor and Graduate Program Director of biomedical engineering at Virginia Commonwealth University (VCU), Richmond, VA, USA. Additionally, he directs the Advanced Signal Processing in Engineering and Neuroscience (ASPEN) Laboratory at VCU. His research interests encompass biomedical signal processing, machine learning, brain-computer interfaces, and neural engineering.
Aleksandra and Dariusz also spoke to prof. Wlodzislaw Duch.
Wlodzislaw Duch is a distinguished figure in neuroinformatics and artificial intelligence. He heads the Neurocognitive Laboratory at the Center of Modern Interdisciplinary Technologies and leads the Neuroinformatics and Artificial Intelligence group at the University Centre of Excellence Dynamics, Mathematical Analysis, and Artificial Intelligence. With a Ph.D. in theoretical physics/quantum chemistry and a D.Sc. in applied math, Duch has made significant contributions to the field. He has held prestigious positions, including the President of the European Neural Networks Society executive committee and fellowships in renowned international associations. Duch has an extensive publication record, authored books, and served on the editorial boards of numerous journals. Additionally, he has held visiting professor positions at esteemed institutions worldwide.
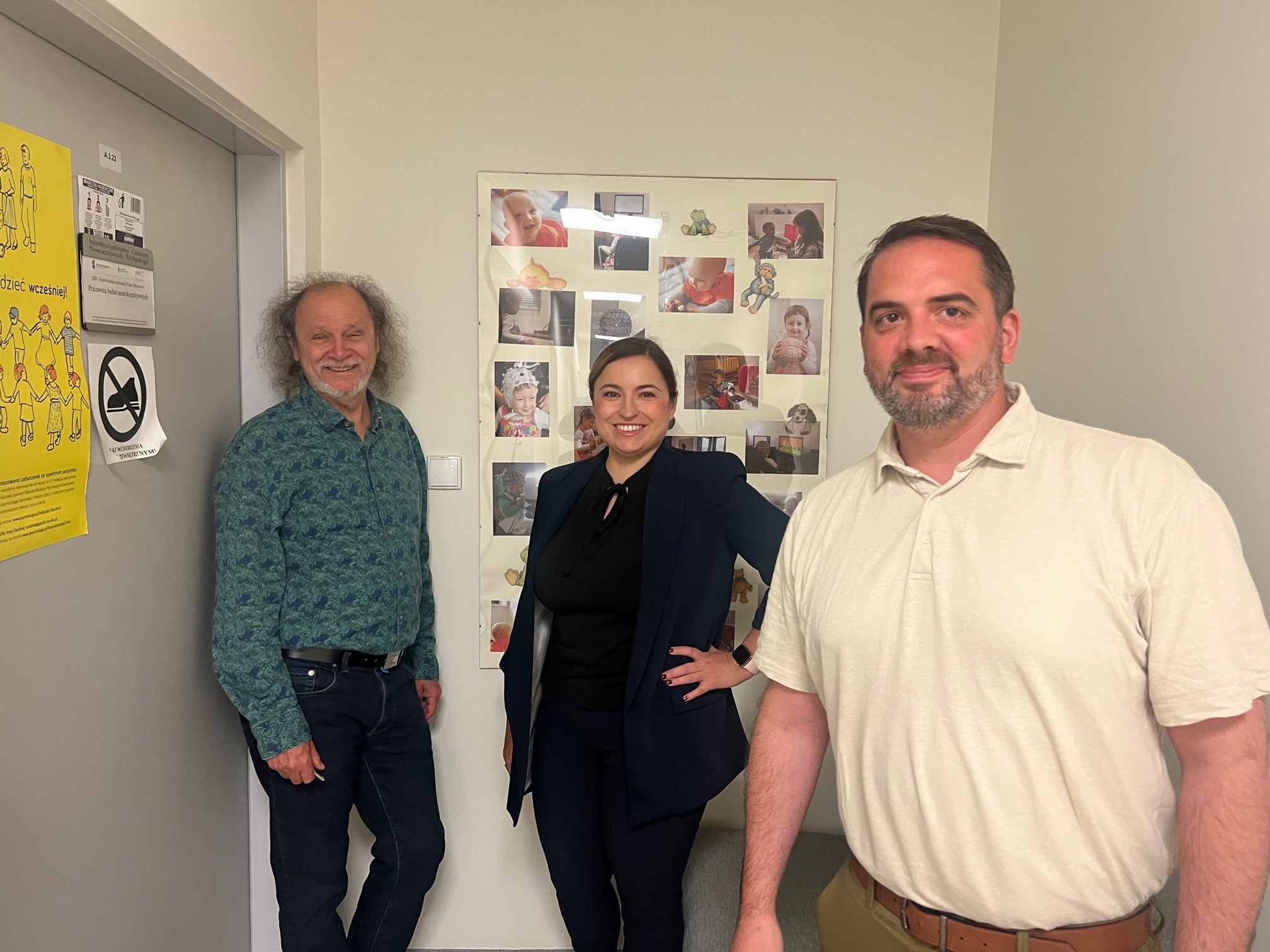
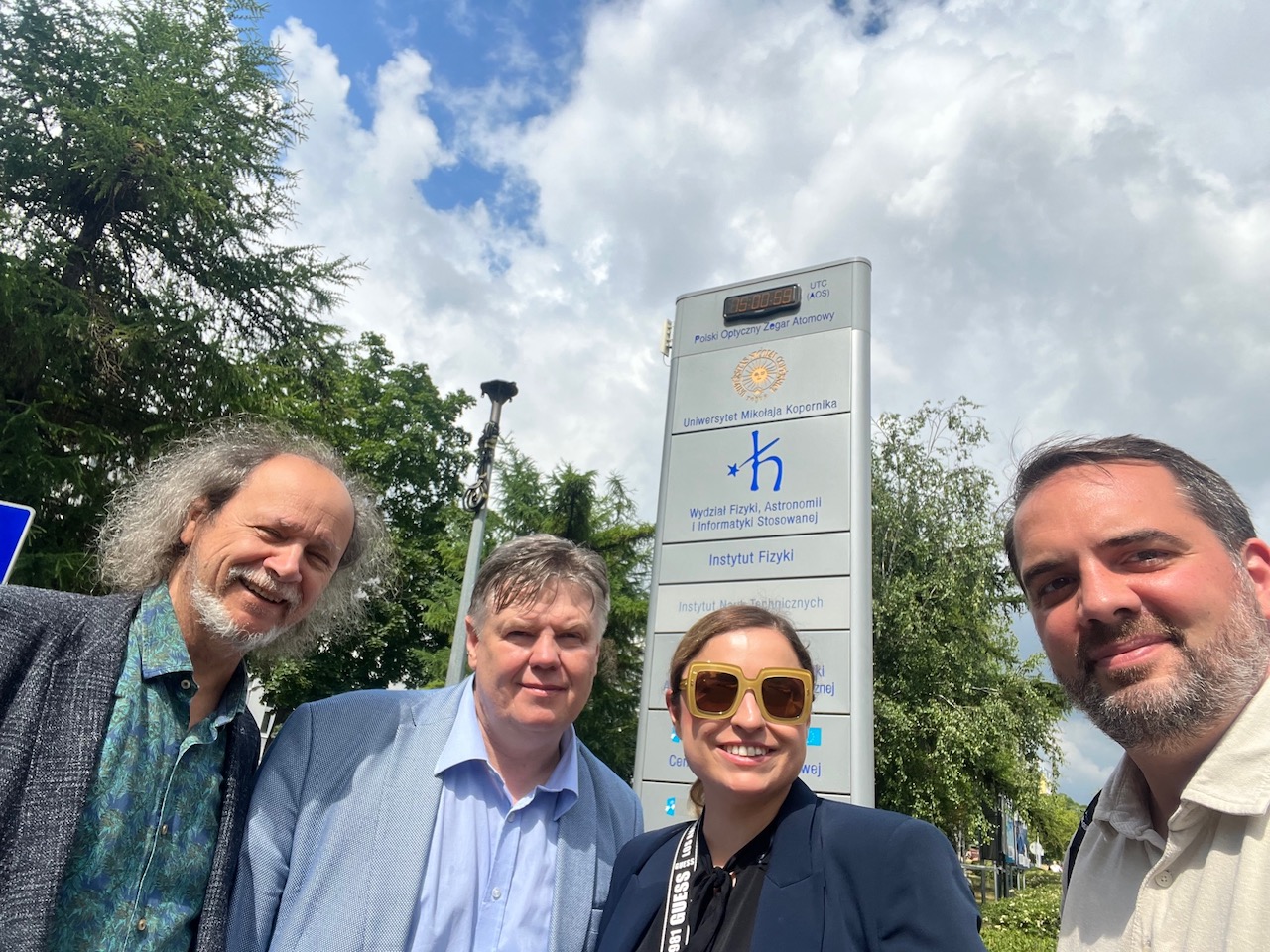
We are looking forward for further cooperation! 🙂